Unlocking the Potential of Labeling Tools for Machine Learning
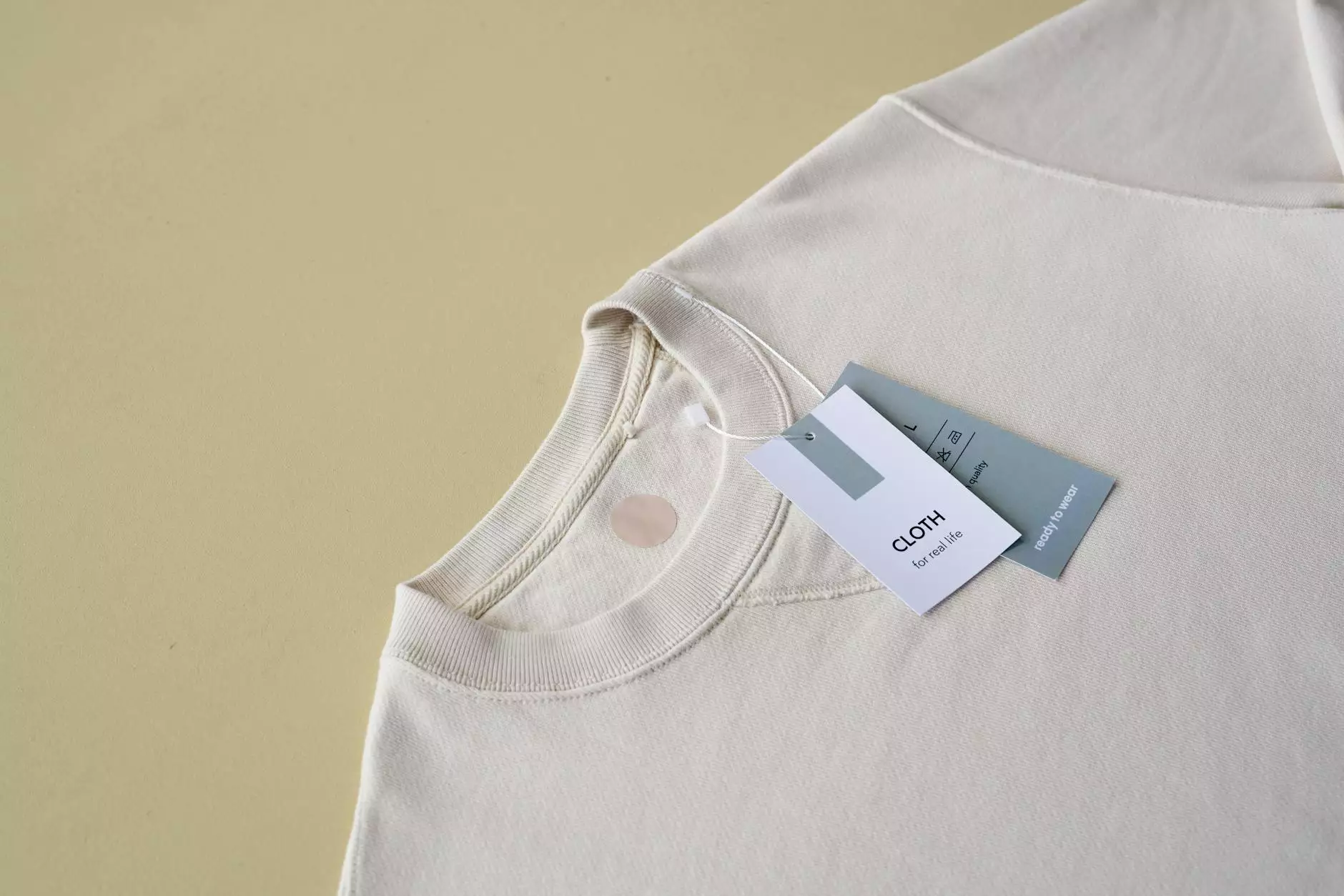
The rise of artificial intelligence and machine learning has transformed various industries, including Home Services, Keys & Locksmiths, and beyond. At the forefront of this revolution are labeling tools for machine learning, which serve as crucial components in training algorithms to recognize patterns and make accurate predictions. In this article, we will delve deeply into what labeling tools are, their significance in machine learning, and how they can enhance business operations.
What Are Labeling Tools?
Labeling tools, also known as data annotation tools, are software applications that enable users to categorize and label datasets which machines can then interpret. These tools are essential in supervised learning—a type of machine learning where algorithms are trained using labeled datasets. The labeled data serves as the guide for the model to learn and make accurate predictions on new, unseen data.
The Importance of Data Annotation
The process of data annotation is integral to machine learning success. A well-annotated dataset can lead to models that perform markedly better, while poorly labeled data can hinder an algorithm's ability to learn effectively. The quality of labeling directly impacts the overall outcome of machine learning projects, particularly in high-stakes industries, including home services and locksmith operations.
Key Benefits of Using Labeling Tools for Machine Learning
- Improved Model Accuracy: High-quality annotations lead to better model predictions, increasing the accuracy of machine learning applications.
- Faster Training Times: Automated and efficient labeling tools streamline the preparation of datasets, significantly reducing time spent on data annotation.
- Cost Efficiency: By leveraging advanced labeling tools, businesses can save on labor costs associated with manual data entry and correction.
- Scalability: As data volumes grow, scalable labeling tools can handle large datasets without sacrificing quality.
- Better Insights: Well-labeled data allows for deeper analysis and better business insights, which can inform strategic decisions.
Choosing the Right Labeling Tool
When it comes to selecting the most suitable labeling tool for machine learning, several factors must be considered:
1. Type of Data
Different projects require different types of data labels. Whether you're dealing with images, text, audio, or video, ensure that the labeling tool you choose specializes in that specific format.
2. User-Friendliness
The tool should offer an intuitive interface that allows users to easily annotate data without extensive training or technical expertise.
3. Automation Capabilities
Look for tools that provide automation features, including pre-labeling and machine learning-assisted tagging, to boost efficiency.
4. Integration with Other Tools
The labeling tool should integrate seamlessly with existing data pipelines, analytics platforms, and machine learning frameworks.
5. Support and Community
A strong support network and an active user community can provide valuable resources, tips, and troubleshooting, making it easier to resolve any challenges that arise.
Top Labeling Tools in the Market
Several labeling tools have gained immense popularity in the machine learning community, known for their features, functionality, and convenience:
1. Labelbox
Labelbox is a leading labeling platform that offers an intuitive interface and supports image, video, and text annotation. Its collaborative capabilities empower teams to annotate efficiently, while its automation features enhance productivity.
2. VGG Image Annotator (VIA)
VIA is an open-source tool for image annotation that is particularly favored for its flexibility and ease of use. It allows users to draw bounding boxes, polygons, and more, making it an excellent choice for various image datasets.
3. Supervisely
Supervisely is another robust tool that supports multiple data types, including images and videos. It provides rich features for annotation, as well as built-in tools for training and deploying machine learning models.
4. Snorkel
Snorkel revolutionizes the labeling process by allowing users to programmatically create labeled training data using weak supervision methods. This approach can dramatically reduce the amount of manual labeling necessary.
5. Prodigy
Prodigy is a modern annotation tool that is highly regarded for its interactivity and efficiency. It incorporates active learning to streamline the annotation process and optimize data quality.
Implementing Labeling Tools in Your Business
For businesses engaged in home services and locksmith operations, leveraging labeling tools for machine learning can provide significant advancements in operational efficiency and customer satisfaction. Here’s how to implement these tools effectively:
1. Identify Business Needs
Clearly outline the objectives of your machine learning project. What do you want to achieve with your labeled data? Understanding the end goal helps in selecting the right tools and annotation strategies.
2. Train Your Team
Ensure that your staff is adequately trained in using the chosen labeling tools. Knowledgeable employees can significantly enhance the quality of data annotation while reducing errors.
3. Establish Quality Control Processes
Implement a robust quality assurance process for labeled data. Regularly review and audit the annotations to maintain high standards and rectify any discrepancies.
4. Leverage Automation
Utilize automation features provided by labeling tools to decrease manual effort and speed up the overall annotation process. This allows your team to focus on more critical aspects of the project.
5. Iterate and Improve
Machine learning is an iterative process. Continue to refine your data annotation efforts based on feedback and performance metrics to enhance model accuracy over time.
The Future of Labeling Tools in Machine Learning
The realm of labeling tools for machine learning is constantly evolving. As machine learning technology advances, we can expect greater integration of automated labeling, enabling even faster data processing and enhanced model training. Moreover, the rise of complex, multi-modal datasets will necessitate sophisticated labeling solutions that are adaptable and efficient.
Emerging Trends
Some notable emerging trends in the field of labeling tools include:
- AI-Assisted Labeling: Improved AI algorithms are making labeling tools more intuitive, allowing them to suggest labels based on previously annotated data.
- Enhanced Collaboration: Cloud-based solutions provide teams with the ability to collaborate in real-time, regardless of geographical location.
- Quality Assurance Automation: New innovations are being developed that automate quality checks, ensuring higher consistency and accuracy in annotated datasets.
Conclusion
In the competitive landscapes of various industries, utilizing the right labeling tools for machine learning can empower businesses to extract valuable insights from their data. As we have seen throughout this article, these tools are not only vital for crafting high-quality datasets but also play a crucial role in achieving superior model performance and operational efficiency. For businesses in the Home Services and Keys & Locksmiths sectors, integrating effective labeling strategies will undoubtedly pave the way for enhanced customer interactions and improved service delivery.
By adopting a thoughtful approach to data annotation and leveraging advanced labeling tools, companies can harness the power of machine learning to drive innovation and stay ahead of the competition.