Medical Image Annotation: Revolutionizing Healthcare Through Precision and Detail
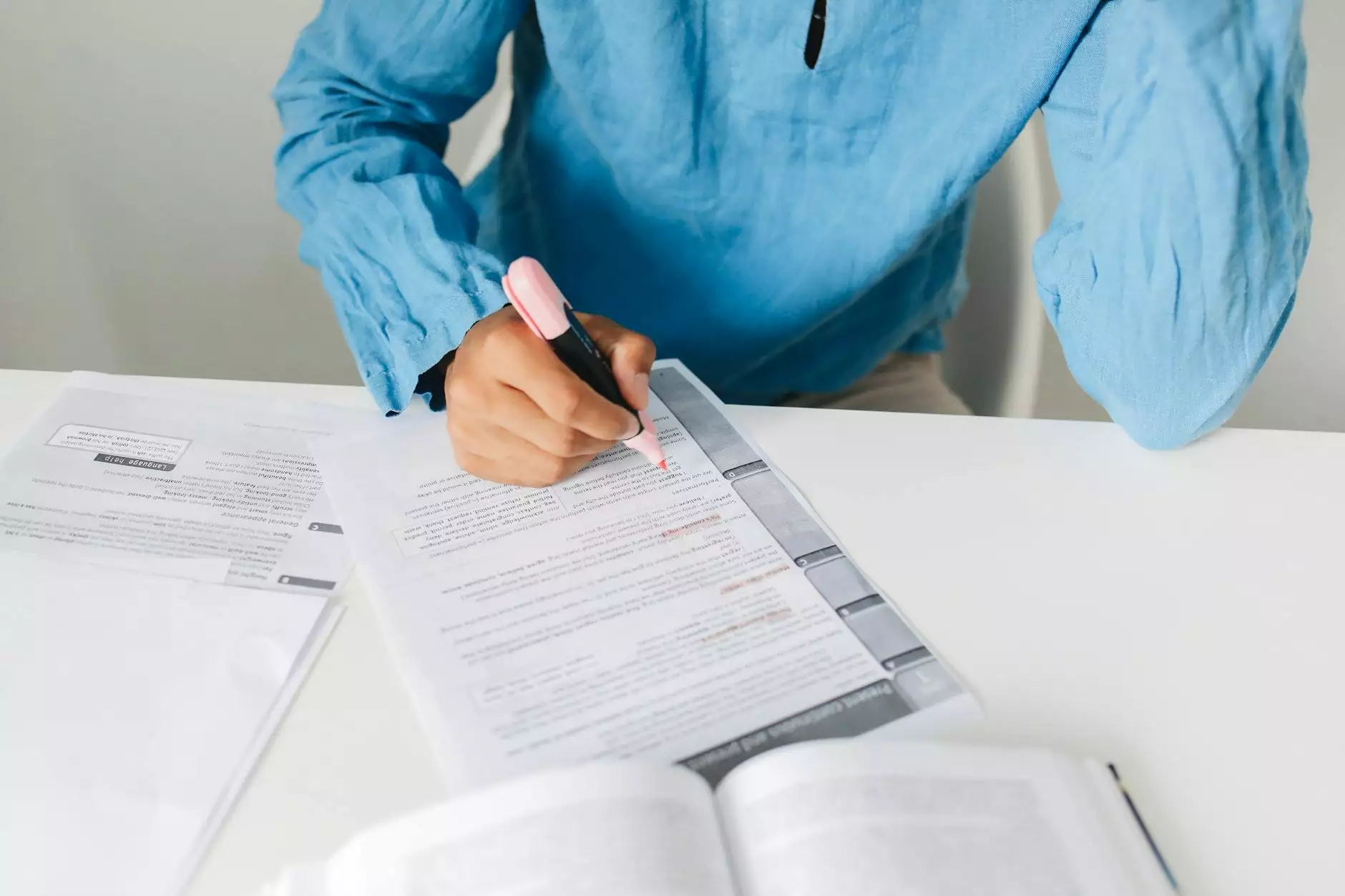
In the contemporary healthcare landscape, the importance of precise and accurate diagnostics cannot be overstated. As medical technologies and methodologies continue to advance, medical image annotation has emerged as a vital component that bridges the gap between raw imaging data and actionable clinical insights. This article delves into the multifaceted world of medical image annotation, highlighting its significance, processes, and impact on patient care and outcomes.
Understanding Medical Image Annotation
Medical image annotation refers to the process of labeling and categorizing images obtained from various medical imaging techniques, such as MRI, CT scans, X-rays, and ultrasounds. This process is essential for training machine learning algorithms that can support diagnostic procedures, analyze abnormal findings, and provide automated assistance to healthcare professionals. By creating annotated datasets, experts can enable artificial intelligence (AI) systems to recognize patterns, detect anomalies, and facilitate timely interventions.
The Importance of Annotation in Healthcare
With the rapid growth of healthcare technology, the need for efficient and accurate diagnosis is more critical than ever. Here are key points highlighting the importance of medical image annotation:
- Improved Diagnostic Accuracy: Accurate annotations help in enhancing the diagnostic capabilities of healthcare providers. AI tools trained on meticulously annotated images can reduce human error and improve overall diagnostic accuracy.
- Streamlined Workflow: By automating aspects of image analysis, medical image annotation allows radiologists and other healthcare professionals to focus on decision-making rather than data processing.
- Enhanced Research Capabilities: Annotated datasets promote advancements in research by providing a substrate for testing new algorithms, thus contributing to the development of better diagnostic tools.
- Informed Treatment Decisions: Through detailed medical image annotations, practitioners can make more informed treatment choices, leading to personalized patient care.
Types of Medical Image Annotation
Medical image annotation can be classified into several types based on the purpose of the annotation:
- Bounding Boxes: These are rectangular boxes drawn around areas of interest in the image, commonly used to highlight tumors or lesions.
- Segmentation: This involves partitioning an image into multiple segments, providing more detailed information and improving the understanding of structures within the imaging data.
- Landmark Annotation: Specific points, or landmarks, are marked on the images, essential for tracking changes over time and assisting in treatment planning.
- Polygonal Annotation: This method uses polygons to enclose irregular shapes, providing precise definitions of regions of interest in complex images.
The Role of Artificial Intelligence in Medical Image Annotation
The integration of artificial intelligence in the medical image annotation process has transformed the way medical imaging is conducted:
AI Algorithms: Deep learning algorithms can analyze vast volumes of medical images quickly and accurately. These systems learn from annotated datasets, becoming capable of identifying patterns that may elude the human eye.
Automation of Workflows: AI can automate the initial stages of image analysis, allowing healthcare professionals to concentrate on interpreting results rather than performing repetitive tasks.
Benefits of AI-Powered Annotation
- Speed: AI algorithms can process and analyze images much faster than human annotators.
- Scalability: Machine learning models can easily scale with the increasing data volume in healthcare.
- Consistency: AI provides consistent results, reducing variability and enhancing reliability in image interpretation.
Challenges in Medical Image Annotation
Despite its advantages, the field of medical image annotation faces significant challenges:
Quality Control:
Maintaining high-quality annotations is paramount. Variability in human annotators’ interpretations can lead to inconsistencies that impact the performance of AI systems. Therefore, rigorous quality control processes are essential.
Data Privacy:
Handling medical image data requires strict adherence to privacy regulations. Ensuring that patient data is anonymized is crucial to comply with laws such as HIPAA (Health Insurance Portability and Accountability Act).
Need for Expertise:
Highly trained medical professionals are required for accurate annotation. This necessitates a significant investment in training and resources.
Implementing Efficient Medical Image Annotation Processes
To harness the full potential of medical image annotation, healthcare organizations can implement the following strategies:
- Adopt Standardized Protocols: Establishing protocols ensures consistency across all imaging procedures and annotation tasks.
- Invest in Training: Continuous training programs for annotators and healthcare professionals are crucial for maintaining high standards of annotation quality.
- Utilize Advanced Tools: Leveraging software tools designed for medical image annotation can streamline processes and improve accuracy.
- Engage in Cross-Disciplinary Collaboration: Collaborating with technologists and data scientists can foster innovations in image processing and analysis.
Future Trends in Medical Image Annotation
Looking ahead, several trends are expected to shape the future of medical image annotation:
- Increased Use of Deep Learning: As deep learning technologies continue to evolve, their application in medical imaging will become more widespread, leading to smarter diagnostic tools.
- Integration with Telemedicine: The rise of telemedicine creates opportunities for remote image annotation, expanding access to expertise.
- Personalized Medicine: Enhanced annotation processes will contribute to personalized treatment plans tailored to individual patient needs.
- Real-time Annotation: Innovations in real-time data processing could lead to live annotations during surgeries and other medical procedures.
Conclusion: The Transformational Impact of Medical Image Annotation
Medical image annotation is not just a technical necessity; it is a transformative force in the healthcare industry. By improving diagnostic accuracy, streamlining workflows, and supporting personalized medicine, it enhances patient care and outcomes significantly. As technology continues to advance, the synergy between medical image annotation and artificial intelligence will unlock new potentials, further revolutionizing how healthcare is delivered. For professionals and organizations in the healthcare industry, embracing medical image annotation is essential to keep pace with ever-evolving medical demands and improve patient outcomes.
For further resources and services related to medical image annotation, visit keymakr.com for comprehensive support in enhancing your business capabilities.